矩陣
由若干行和列元素排列成的矩形陣列 来自维基百科,自由的百科全书
數學上,一個的矩陣(英語:matrix)是一個有列(row)行(column)元素的矩形陣列。矩陣裡的元素可以是數字或符號甚至是函數。
「m-by-n matrix」的各地常用名稱 | |
---|---|
中國大陸 | 行列矩陣 |
臺灣 | 列行矩陣 |
「橫排(row)」的各地常用名稱 | |
---|---|
中國大陸 | 行 |
臺灣 | 列 |
「縱排(column)」的各地常用名稱 | |
---|---|
中國大陸 | 列 |
臺灣 | 行 |
大小相同(行數列數都相同)的矩陣之間可以相互加減,具體是對每個位置上的元素做加減法。矩陣乘法則較為複雜。兩個矩陣可以相乘,當且僅當第一個矩陣的行數等於第二個矩陣的列數。矩陣乘法滿足結合律和分配律,但不滿足交換律。
矩陣的一個重要用途是解線性方程組。線性方程組中未知量的係數可以排成一個矩陣,加上常數項,則稱為增廣矩陣。另一個重要用途是表示線性變換,即是諸如之類的線性函數的推廣。設定基底後,某個向量可以表示為的矩陣,而線性變換可以表示為行數為的矩陣,使得經過變換後得到的向量可以表示成的形式。矩陣的特徵值和特徵向量可以揭示線性變換的深層特性。
矩陣是高等代數學中的常見工具,也常見於統計分析等應用數學學科中。在物理學中,矩陣在力學、電路學、光學和量子物理等領域中都有應用;計算機科學中,三維動畫製作也需要用到矩陣。矩陣的運算是數值分析領域的重要問題。將矩陣分解為簡單矩陣的組合可以在理論和實際應用上簡化矩陣的運算。對一些應用廣泛而形式特殊的矩陣,例如稀疏矩陣和准對角矩陣,有特定的快速運算算法。關於矩陣相關理論的發展和應用,請參考矩陣理論。在天體物理、量子力學等領域,也會出現無窮維的矩陣,是矩陣的一種推廣。
詞源
中文中矩陣的概念最早見於1922年。1922年,北京師範大學附屬中學數學老師程廷熙在一篇介紹文章中將矩陣譯為「縱橫陣」。1925年,在科學名詞審查會算學名詞審查組刊登於《科學》第十卷第四期的審定名詞表中,矩陣被翻譯為「矩陣式」,方塊矩陣翻譯為「方陣式」,而各類矩陣如「正交矩陣」、「伴隨矩陣」中的「矩陣」則被翻譯為「方陣」。1935年,中國數學會審查後,中華民國教育部審定的《數學名詞》(並「通令全國各院校一律遵用,以昭劃一」)中,「矩陣」作為譯名首次出現。1938年,曹惠群在接受科學名詞審查會委託就數學名詞加以校訂的《算學名詞彙編》中,認為應當的譯名是「長方陣」。1949年中華人民共和國成立後編訂的《數學名詞》中,則將譯名定為「(矩)陣」。1993年,中國自然科學名詞審定委員會公布的《數學名詞》中,「矩陣」被定為正式譯名,並沿用至今[1]。
發展
总结
视角
作為解決線性方程的工具,矩陣也有不短的歷史。成書最遲在東漢前期的《九章算術》中,已經出現過以矩陣形式表示線性方程組係數以解方程的圖例,可視為矩陣的雛形[2]。矩陣正式作為數學中的研究對象出現,則是在行列式的研究發展起來後。邏輯上,矩陣的概念先於行列式,但在歷史上則恰好相反。日本數學家關孝和(1683年)與微積分的發現者之一戈特弗里德·威廉·萊布尼茨(1693年)近乎同時獨立建立了行列式論。其後行列式作為解線性方程組的工具逐步發展。1750年,加布里爾·克拉默發現了克萊姆法則[3]。

進入十九世紀後,行列式的研究進一步發展,矩陣的概念也應運而生。奧古斯丁·路易·柯西是最早將行列式排成方陣並將其元素用雙重下標表示的數學家。他還在1829年就在行列式的框架中證明了實對稱矩陣特徵根為實數的結論[4]。其後,詹姆斯·約瑟夫·西爾維斯特注意到,在作為行列式的計算形式以外,將數以行和列的形式作出的矩形排列本身也是值得研究的。在他希望引用數的矩形陣列而又不能用行列式來形容的時候,就用「matrix」一詞來形容[3]。而在此之前,數學家已經開始將增廣矩陣作為獨立的對象引用了。西爾維斯特使用「matrix」一詞是因為他希望討論行列式的子式,即將矩陣的某幾行和某幾列的共同元素取出來排成的矩陣的行列式,所以實際上「matrix」被他看做是生成各種子式的「母體」:
我在先前的文章中將矩形排布的序列稱為「Matrix」,蓋因從中可以產生出各種不同的行列式,就如由同一個母體的子宮中孕育出來一樣。[5]
阿瑟·凱萊被公認為矩陣論的奠基人[3]。他開始將矩陣作為獨立的數學對象研究時,許多與矩陣有關的性質已經在行列式的研究中被發現,這也使得凱萊認為矩陣的引進是十分自然的。他說:「我決然不是通過四元數而獲得矩陣概念的;它或是直接從行列式的概念而來,或是作為一個表達線性方程組的方便方法而來的。[3]」他從1858年開始,發表了《矩陣論的研究報告》等一系列關於矩陣的專門論文[6][7],研究了矩陣的運算律、矩陣的逆以及轉置和特徵多項式方程。凱萊還提出了凱萊-哈密爾頓定理,並驗證了3×3矩陣的情況,又說進一步的證明是不必要的。哈密爾頓證明了4×4矩陣的情況,而一般情況下的證明是弗羅貝尼烏斯於1898年給出的[3]。
此後更多數學家開始對矩陣進行研究。埃爾米特證明了如果矩陣等於其復共軛轉置,則特徵根為實數。這種矩陣後來被稱為埃爾米特矩陣[3]。弗羅貝尼烏斯對矩陣的特徵方程、特徵根、矩陣的秩、正交矩陣、矩陣方程等方面做了大量工作。1878年,在引進了不變因子、初等因子等概念的同時,弗羅貝尼烏斯給出了正交矩陣、相似矩陣和合同矩陣的概念。同年,他探討了矩陣的最小多項式(最小方程)問題。1894年的論文中,他討論了矩陣理論和四元數理論的關係。1896年,他給出了凱萊-哈密爾頓定理的完整證明[1]。矩陣理論在19世紀沿着兩個方向發展,分別是作為抽象代數結構和作為代數工具描述幾何空間的線性變換。矩陣理論為群論和不變量理論的發展。
無限維矩陣的研究始於1884年。龐加萊在兩篇不嚴謹地使用了無限維矩陣和行列式理論的文章後開始了對這一方面的專門研究[1]。1906年,希爾伯特引入無限二次型(相當於無限維矩陣)對積分方程進行研究,極大地促進了無限維矩陣的研究。在此基礎上,施密茨、赫林格和特普利茨發展出算子理論,而無限維矩陣成為了研究函數空間算子的有力工具[1]。
正式定義
矩陣的定義 — 是一個集合,那函數 就會被稱為定義在 上的 矩陣
直觀上就是用兩個數碼去標記一堆數學實體(如數字、函數),實際上是有限序列的一種推廣。
被暱稱為矩陣 裡的元素,通常簡記為、或。除此之外也會用小寫字母 表示元素,來跟矩陣 本身做區別。但不知 的具體形式卻想強調 為 的元素的話,可以 或 表示。
如果表達式 (嚴格來說是合式公式,其中 為一個包含變數 的項)可以唯一決定一個矩陣 ,那會將它記成 。如:
- 且
就可以表達為 。
根據公理化集合論,可以定義一個函數的集合 ,它囊括所有定義在 上的 矩陣,也就是說:
以下的 矩陣:
一般會如下排列成為矩形來表示:
英文將橫向的元素組統稱為「row」,縱向統稱為「column」;但兩岸對此卻以不同的稱呼;在中國大陸,橫向的元素組稱為「行」,縱向稱為「列」,而在臺灣則相反,橫向稱為「列」,縱向稱為「行」[8]。
是矩陣
的簡寫。要注意的是,計算機編程中,由於數組的首項是第0項,故編程者可能會將第1行/列稱為第0行/列,從而對矩陣的寫法產生影響,比如矩陣就要改寫成。
矩陣的元素可以是數字、符號或數學表達式。一般為了支援矩陣的運算,矩陣的元素之間應當能做加減法和乘法,所以是某個環裡的元素。最常見的是元素屬於實數域或複數域的矩陣,簡稱為實矩陣和復矩陣。更一般的情況下,矩陣的元素可以是由一個環中的元素排成。給定一個環,所有由中元素排成的矩陣的集合寫作或。若,則通常記以或,稱其為維矩陣或方陣。
矩陣的基本運算
矩陣的最基本運算包括矩陣加(減)法,數乘和轉置運算。被稱為「矩陣加法」、「數乘」和「轉置」的運算不止一種[9],其中最基本最常用的定義如下:
運算 | 定義 | 例子 |
---|---|---|
加(減)法 | 矩陣和的和(差):為一個矩陣,其中每個元素是和相應元素的和(差),
|
|
數乘 | 標量與矩陣的數乘:的每個元素是的相應元素與的乘積,
|
|
轉置 | 矩陣的轉置是一個的矩陣,記為(有些書中也記為或、),其中的第個列向量是原矩陣的第個行向量;或者說,轉置矩陣第列第行的元素是原矩陣第列第行的元素,
|
矩陣的加法運算滿足交換律:[10]。矩陣的轉置和數乘運算對加法滿足分配律:
矩陣加法和數乘兩種運算使得成為一個維的實數線性空間。而轉置和數乘運算滿足類似於結合律的規律:
矩陣也有類似行列式的初等變換,即對矩陣的某些行和某些列進行三類操作:交換兩行/列,將一行/列的每個元素都乘以一個固定的量,以及將一行/列的每個元素乘以一個固定的量之後加到另一行/列的相應元素上。這些操作在求其逆矩陣時有用。
矩陣乘法
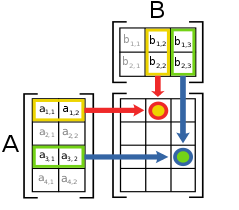
兩個矩陣的乘法僅當第一個矩陣的行數(column)和另一個矩陣的列數(row)相等時才能定義。如是矩陣和是矩陣,它們的乘積是一個矩陣,它的一個元素
其中'[11]。
例如
矩陣的乘法滿足結合律和對矩陣加法的分配律(左分配律和右分配律):
- 結合律:
- 左分配律:
- 右分配律:
矩陣的乘法與數乘運算之間也滿足類似結合律的規律;與轉置之間則滿足倒置的分配律。
矩陣乘法不滿足交換律。一般來說,矩陣及的乘積存在,但不一定存在,即使存在,大多數時候。比如下面的例子:
。
這一特性使得矩陣代數與常見的一些數域(有理數、實數、複數)以及環(多項式環、整數環)都不同。給定一個維的方塊矩陣,與交換的所有方塊矩陣構成一個環,稱為的交換子環。這些矩陣也構成的一個子空間,稱為的可交換空間[12]。與中所有矩陣交換的矩陣只有形如的矩陣(稱為數乘矩陣)。其中的是單位矩陣,也就是主對角線上的元素為1,其它元素為0的矩陣。任意矩陣乘以單位矩陣都得到自身:。
矩陣乘法的一個基本應用是在線性方程組上。線性方程組是方程組的一種,它符合以下的形式:
其中的以及等等是已知的常數,而等等則是要求的未知數。運用矩陣的方式,可以將線性方程組寫成一個向量方程:
其中,是由方程組裡未知量的係數排成的矩陣,是含有個元素的列向量,是含有個元素的列向量[14]。
這個寫法下,將原來的多個方程轉化成一個向量方程,在已知矩陣和向量的情況下,求未知向量。
矩陣是線性變換的便利表達法。矩陣乘法的本質在聯繫到線性變換的時候最能體現,因為矩陣乘法和線性變換的合成有以下的聯繫: 以表示所有長度為的列向量的集合。每個的矩陣都代表了一個從射到的線性變換。反過來,對每個線性變換,都存在唯一m×n矩陣使得對所有中的元素,。這個矩陣第列第行上的元素是正則基向量(第j個元素是1,其餘元素是0的向量)在映射後的向量的第個元素。
也就是說,從射到的線性變換構成的向量空間上存在一個到的一一映射:
以下是一些典型的2維實平面上的線性變換對平面向量(圖形)造成的效果,以及它們對應的2維矩陣。其中每個線性變換將藍色圖形映射成綠色圖形;平面的原點(0, 0)用黑點表示。
推移, 幅度m=1.25. |
水平鏡射變換 | 「擠壓」變換, 壓縮程度r=3/2 |
伸縮,3/2倍 | 旋轉,左轉30° |
![]() |
![]() |
![]() |
![]() |
![]() |
設有的矩陣代表線性變換,則矩陣積代表了綫性變換的複合[15],因為
矩陣的秩是指矩陣中線性無關的行/列向量的最大個數[16],同時也是矩陣對應的線性變換的像空間的維度[17]。秩-零化度定理說明矩陣的行數量等於矩陣的秩與零空間維度之和[18]。
方塊矩陣
行數與列數相同的矩陣稱為方塊矩陣,簡稱方陣。所有維的方塊矩陣構成一個線性空間,這個空間對矩陣乘法也是封閉的,因此也是一個代數。方陣稱為可逆或非奇異的,如果存在另一個方陣,使得
成立。這時候可以證明也有成立[19],可將矩陣稱為的逆矩陣[20]。一個矩陣的逆矩陣如果存在的話,就是唯一的,通常記作。
矩陣的元素稱為其主對角線上的元素。方塊矩陣的所有主對角線元素之和稱為它的跡,寫作。儘管矩陣的乘法不滿足交換律,方陣相乘時交換順序會導致乘積變化,但它們的跡不會變,即[21]。除此以外,矩陣轉置的跡等於其自身的跡,。
如果一個方陣只有主對角線上的元素不是0,其它都是0,那麼稱其為對角矩陣。如果主對角線上方的元素都是0,那麼稱為下三角矩陣;反之如果主對角線下方的元素都是0,那麼稱為上三角矩陣。例如的時候,這些矩陣分別寫作:
- (對角矩陣),(下三角矩陣)和(上三角矩陣)。

方塊矩陣的行列式是一個將其映射到標量的函數,記作或,反映了矩陣自身的一定特性。一個方陣的行列式等於0當且僅當該方陣不可逆。係數是實數的時候,二維(三維)方陣的行列式的絕對值表示單位面積(體積)的圖形經過對應的線性變換後得到的圖形的面積(體積),而它的正負則代表了對應的線性變換是否改變空間的定向:行列式為正說明它保持空間定向,行列式為負則說明它逆轉空間定向。
2×2矩陣的行列式是
- 。
3×3矩陣的行列式由6項組成。更高維矩陣的行列式則可以使用萊布尼茲公式寫出[22],或使用拉普拉斯展開由低一維的矩陣行列式遞推得出[23]。
兩個矩陣相乘,乘積的行列式等於它們的行列式的乘積:[24]。將矩陣的一行/列乘以某個係數加到另一行/列上不改變矩陣的行列式,將矩陣的兩行/列互換則使得其行列式變號[25]。用這兩種操作可以將矩陣變成一個上三角矩陣或下三角矩陣,而後兩種矩陣的行列式就是主對角線上元素的乘積,因此能方便地計算。運用行列式可以計算線性方程組的解(見克萊姆法則)[26]。
的方塊矩陣的一個特徵值和對應特徵向量是滿足
- [27]的標量以及非零向量。特徵值和特徵向量的概念對研究線性變換很有幫助。一個線性變換可以通過它對應的矩陣在向量上的作用來可視化。一般來說,一個向量在經過映射之後可以變為任何可能的向量,而特徵向量具有更好的性質[28]。假設在給定的基底下,一個線性變換對應着某個矩陣,如果一個向量可以寫成矩陣的幾個特徵向量的線性組合:
其中的表示此向量對應的特徵值是,那麼向量經過線性變換後會變成:
可以清楚地知道變換後向量的結構。
另一個等價的特徵值定義是:標量為特徵值,如果矩陣是不可逆矩陣。根據不可逆矩陣的性質,這個定義也可以用行列式方程描述:為特徵值,如果
- [29]這個定義中的行列式可以展開成一個關於的n階多項式,叫做矩陣A的特徵多項式,記為。特徵多項式是一個首一多項式(最高次項係數是1的多項式)。它的根就是矩陣特徵值[30]。哈密爾頓-凱萊定理說明,如果用矩陣本身代替多項式中的不定元,那麼多項式的值是零矩陣[31]:
。
轉置等於自己的矩陣,即滿足的方塊矩陣叫做對稱矩陣。滿足的矩陣稱為反對稱矩陣。在復係數矩陣中,則有埃爾米特矩陣的概念:滿足的方塊矩陣稱為埃爾米特矩陣,其中的表示的共軛轉置矩陣。
根據譜定理,實對稱矩陣和復埃爾米特矩陣擁有特徵基,即由矩陣的特徵向量組成的基底。因此任何向量都能表示成矩陣特徵向量的線性組合。此外,這兩類矩陣的特徵值都是實數[32]。
矩陣表達式 | ||
正定性 | 不定矩陣 | 正定矩陣 |
對應二次型 | ||
取值圖像 | ![]() | |
說明 | 正定矩陣對應的二次型的取值範圍永遠是正的, 不定矩陣對應的二次型取值則可正可負 |
的實對稱矩陣如果滿足對所有非零向量,對應的二次型
函數值都是正數,就稱為正定矩陣。類似地還有半正定矩陣、負定矩陣、不定矩陣等概念[33]。對稱矩陣的正定性與其特徵值密切相關。矩陣是正定的當且僅當其特徵值都是正數[34]。
矩陣的計算
矩陣在許多學科領域中都有應用,在很多時候,除了需要知道矩陣的理論性質以外,還需要計算矩陣的數值。為了矩陣的計算能夠足夠精確與快捷,數值線性代數中專門有研究矩陣的數值計算方法[35]。與其它的數值計算一樣,矩陣的數值計算注重的主要也是算法的複雜度和數值穩定性。矩陣的數值計算可以使用直接計算,也可以用迭代算法,例如在計算方塊矩陣的特徵值時,可以從一個非零向量開始,通過特定迭代方法得到一個逼近某個特徵向量的向量序列[36]。
測量一個算法的複雜度是指估計此算法需要的基本運算如數字的加法和乘法的次數,或者找出它的一個上界。例如按照定義計算的話,兩個階方陣的乘法需要次數字乘法計算,因為其乘積是一個階方陣,有個元素,計算每個元素需要次數字乘法。如果使用施特拉森算法的話,可以將數字乘法的次數減低到大約次[37]。此外,編程語言或環境本身對算法的複雜度也會有影響。
某些特殊類型的矩陣攜帶的數據量比一般矩陣要少,同時帶來的信息量比一般矩陣多。一個重要的例子是稀疏矩陣,這類矩陣中絕大部分的元素是零。有關稀疏矩陣的計算,如計算稀疏矩陣的線性方程組時,可以使用一些專用於稀疏矩陣的特殊算法(比如共軛梯度法[38]),減低計算複雜度。
算法的數值穩定性是指輸入值的小變化不會讓計算結果產生很大偏差。例如計算矩陣的逆時,可以用以下的算法(其中表示的伴隨矩陣,表示的行列式)
這個算法在的行列式接近0的時候會引起很大的捨入誤差[39]。而如果使用全選主元的高斯消去法求逆,則在複雜度降低的同時能夠避免捨入誤差,保證數值穩定性。
矩陣研究的一大方向是將一般的矩陣用一些比較「簡單」的矩陣來表示。這種表示方式稱為矩陣的變換與分解。矩陣變換與分解的方法有很多,它們的目的都是希望化簡後的矩陣保持原矩陣的某些性質,比如行列式、秩或逆矩陣,而形式相對簡單,因而能用容易地進行討論和計算,或者能使得某些算法更易執行。
LU分解將矩陣分解為一個下三角矩陣和一個上三角矩陣的乘積[40]。分解後的矩陣可以方便某些問題的解決。例如解線性方程組時,如果將係數矩陣分解成的形式,那麼方程的求解可以分解為求解和兩步,而後兩個方程可以十分簡潔地求解(詳見三角矩陣中「向前與向後替換」一節)。又例如在求矩陣的行列式時,如果直接計算一個矩陣的行列式,需要計算大約次加法和乘法;而如果先對矩陣做分解,再求行列式,就只需要大約次加法和乘法,大大降低了計算次數。這是因為做分解的複雜度大約是次,而後注意到和是三角矩陣,所以求它們的行列式只需要將主對角線上元素相乘即可。

高斯消去法也是一種矩陣分解方法。通過初等變換操作,可以將任何矩陣變為階梯形矩陣,而每個操作可以看做是將矩陣乘上一個特定的初等矩陣[41]。奇異值分解則是另一種分解方法,將一個矩陣表示成3個矩陣的乘積:。其中和是酉矩陣,是對角矩陣。
特徵分解是將一個矩陣寫成的形式,其中是一個可逆矩陣,是對角矩陣[42]。如果的特徵分解存在,就稱它是可對角化的矩陣。不能對角化的矩陣,也有類似的分解方式。任意的矩陣都可以寫成的形式,其中的矩陣是若爾當標準型。若爾當標準型是矩陣的一種,它與對角矩陣類似,只不過主對角線上的元素不是數值,而是若爾當塊:主對角線上為同一元素,主對角線右上一行的次對角線上都是1,其它元素都是0的矩陣(見右圖)[43]。特徵分解可以方便計算矩陣的冪次和多項式,如要計算:
而其中對角矩陣的冪次要比容易計算得多。同理還可計算矩陣指數:(在線性微分方程中有應用)、矩陣對數和矩陣的平方根[44]。為了提高算法的數值穩定性,還有舒爾分解等矩陣分解方法[45]。
矩陣的推廣
矩陣的元素除了可以是實數和複數以外,也可以任意環或域中元素。在線性代數中,矩陣的性質可以經由有限維的線性空間中的線性變換定義。更廣泛的,無限維空間中的線性算子,則可以定義更廣泛的無窮維矩陣。矩陣的另一種推廣是張量。標量可以看成零維方式排列的數據(只有一個「點」),向量可以看成是一維方式排列的數據(若干個「點」排成的「線段」),矩陣可以看成是二維方式排列的數據(若干個「線段」排成的「矩形」),而張量的概念則包括了這幾種排列方式。在張量的概念中,標量是零維張量,向量是一維張量,矩陣是二維張量,而更高維方式排列的數據方式就是高維張量[46]。
矩陣的元素除了可以是實數和複數以外,還可以是任何能夠使得矩陣的運算律成立的元素。首先,矩陣的元素可以是任意一個域(即能夠進行「加減乘除」運算的集合)中元素。例如編碼理論中會出現係數為有限域中元素的矩陣,以及有理數係數的矩陣。如果矩陣的係數所在域不是代數閉域,那麼在求矩陣的特徵值時,由於特徵值是相應的特徵多項式的根,可能不在係數域中,而是在係數域的某個擴域L中。反過來,如果考慮擴域,以及中的一個元素,以及中線性變換,那麼由於也是一個-線性變換,它可以表示成一個的係數矩陣,其中的是擴域的階數。是這個矩陣的特徵值,這個矩陣的特徵多項式是在中的最小多項式的冪次:
- 。其中的是擴域 的階數[47]。
更一般的情況是矩陣的元素屬於某個環[48]。環是比域更廣泛的概念,只要求其中元素能夠進行加減法和乘法運算(不一定能定義除法)。給定一個環,中的矩陣之間可以相互加減以及相乘,所以關於矩陣的加法和乘法也構成一個環,稱為矩陣環。維方陣的環與左-模的自同態環同構[49]。
若是交換環,則是一個帶單位元的-代數,滿足結合律,但不滿足交換律。其中的矩陣仍然可以用萊布尼茲公式定義行列式。一個矩陣可逆當且僅當其行列式為環中的可逆元(域上的矩陣可逆只需行列式不等於0)[50]。
前面已經提到,所有的線性變換都對應着一個中的矩陣。更一般地,給定了基底後,任意兩個有限維線性空間之間的線性映射也對應着一個矩陣。設空間和的基底分別是和,那麼
- 對任意 ,
矩陣實際上「記錄」了中每個基底向量經過變換後得到的中的像在基底下的形式。要注意矩陣的內容取決於基底的選擇。可以說,矩陣是線性變換f在特定「角度」(基底)下的「素描」。不同的「角度」下,描述的矩陣是不同的,但這些矩陣都是相似矩陣[51]。與矩陣有關的基本概念都可以用線性變換的層面來解釋,比如一個矩陣的轉置可以用f的對偶變換來表示[52]。
當矩陣的元素是帶單位元的環中的元素時,的-矩陣對應的則是-自由模和之間的-線性變換。的時候,這些-線性變換可以相互複合,因此維的-矩陣環能夠與-自同態環同構。
群是比環更寬泛的代數結構,只需要集合配備一個滿足結合律的二元運算,即將兩個群內元素映射到群內一元素的運算。矩陣群是指矩陣關於矩陣乘法組成的群[53]。顯然,只有方塊矩陣才能構成乘法群。所有維的可逆方陣構成一個群,稱為階一般線性群。由於群內每個元素都必須是可逆的,任意的矩陣群都必然是一般線性群的子群。
能夠在矩陣乘法和求逆矩陣運算下保持的性質都可以用來刻畫一定的矩陣群。例如所有行列式為1的矩陣可以構成一個群,稱為階特殊線性群[54]。所有維的正交矩陣,即滿足:
的矩陣也構成一個群,稱為階正交群[55]。正交矩陣得名於它在中對應的線性變換具有保角性,也就是說對基本的點積,滿足
每個有限群都同構於一個矩陣群。實際上,每個有限群都同構於某個置換群的子群,而每個置換群都同構於一個矩陣群(見置換群的正則群表示[57])鑑於矩陣群的性質可以通過與矩陣相關的更多手段更好地理解,常常通過研究矩陣群來研究一個有限群。相關的理論稱為群表示論。
無窮維矩陣可以指行數或列數無窮大,或兩者都是無窮大的矩陣[58]。儘管這樣的矩陣無法完整寫出,但只要知道每行每列的元素的值,仍然可以對它進行矩陣操作和運算。這裡矩陣的行數和列數甚至不一定需要是可數集。需要注意的是,無窮維矩陣的乘法涉及到無窮級數求和,因此只有在相關的無窮級數收斂的時候,才能定義矩陣的乘積[59]。無限維矩陣也可以是方塊矩陣,定義為行標記集合與列標記集合相同的矩陣(如)[60]。
無限矩陣無法定義通常意義上的行列式,因此可逆矩陣不一定是方塊矩陣,同理,酉矩陣也不一定要是方塊矩陣[61]。
空矩陣是指行數或列數為零的矩陣。[62][63]空矩陣的定義可以完善一些關於零維空間的約定。包括約定一個矩陣與空矩陣相乘得到的也是空矩陣,兩個和的空矩陣相乘是一個的零矩陣(所有元素都是零的矩陣)。0×0的空矩陣的行列式約定為1,所以它也可以有逆矩陣,約定為它自己[64]。
分塊矩陣是指一個大矩陣分割成「矩陣的矩陣」。舉例,以下的矩陣
可分割成4個2×2的矩陣
- 。將矩陣分塊可以使得矩陣結構清晰,在某些時候可以方便運算、證明。兩個大小相同、分塊方式也相同的矩陣可以相加。行和列的塊數符合矩陣乘法要求時,分塊矩陣也可以相乘。將矩陣分塊相乘的結果與直接相乘是一樣的。用分塊矩陣求逆,可以將高階矩陣的求逆轉化為多次低階矩陣的求逆[65]。
應用
矩陣在許多領域都應用廣泛。有些時候用到矩陣是因為其表達方式緊湊,例如在博弈論和經濟學中,會用收益矩陣來表示兩個博弈對象在各種決策方式下的收益[66]。文本挖掘和索引典匯編的時候,比如在TF-IDF方法中,也會用到文件項矩陣來追蹤特定詞彙在多個文件中的出現頻率[67]。
複數可以用實係數的2×2矩陣表示:
這種表示法與複數的加減法、乘法都相兼容。比如,2×2的旋轉矩陣可以用來表示模長為1的複數,一個向量乘以此旋轉矩陣可以視作一個複數乘以該模長為1的複數。對四元數也有類似的矩陣表達[68]。
早期的密碼技術如希爾密碼也用到矩陣。然而,矩陣的線性性質使這類密碼相對容易破解[69]。計算機圖像處理也會用到矩陣來表示處理對象,並且用放射旋轉矩陣來計算對象的變換,實現三維對象在特定二維屏幕上的投影[70]。多項式環上的矩陣在控制論中有重要作用。
化學中也有矩陣的應用,特別在使用量子理論討論分子鍵和光譜的時候。具體例子有解羅特漢方程時用重疊矩陣和福柯矩陣來得到哈特里-福克方法中的分子軌道。

圖論中可以用矩陣描述一個有限圖[71]。這個矩陣叫做相關矩陣的鄰接矩陣,記錄了圖的每兩個頂點之間是否有邊連接。對簡單圖來說,鄰接矩陣的元素只取兩個值:0和1,第列第行上取值為0,表示沒有從第個頂點連到第個頂點的邊,取值為1則說明有。如果是一般情況的話,第列第 行上的取值是從第個頂點連到第j個頂點的邊的數目。距離矩陣則是表示圖中各頂點之間距離的矩陣[72]。在研究互聯網等複雜網絡的時候,鄰接矩陣常常會是稀疏矩陣。因此網絡理論中有專門研究稀疏矩陣的方面。
在多元函數微積分學中,對二階偏導數存在的函數,可以定義其海森矩陣[73]:
- 。

嚴格來說,僅當函數在某一點上的二階偏導數存在,才能定義這一點上的海森矩陣。海森矩陣給出了函數在這一點的變化率方面的信息。當給定的點是函數平穩點(即函數在這一點上的一階偏導數都是0)時,就需要利用海森矩陣來查看函數在這一點周圍的增長特性。多元函數在點的泰勒展開是:
如果函數在點x的一階偏導數都是0,那麼,所以函數在x附近的變化率取決於海森矩陣的性質。如果是正定矩陣,那麼函數在點x取得局部最小值,如果是負定矩陣,則函數在x取得局部最大值。在這類情況下,關於函數f的條件最優化問題可以轉變為關於海森矩陣的二次規劃問題[74]。
矩陣在多元函數微積分中的另一個應用是雅可比矩陣。函數在某一點x上的一階偏導數存在時,可以定義它在這點上的雅可比矩陣[75]:
偏微分方程理論中,二階擬線性偏微分方程可以根據最高次偏導項係數構成的矩陣的正定性分類。假設有一個二階擬線性偏微分方程:
- 並假設
記矩陣。如果矩陣是正定或負定矩陣,那麼就稱方程為橢圓形偏微分方程;如果不可逆,就稱為拋物形偏微分方程,如果可逆而且恰有個特徵值同號,就稱為雙曲型偏微分方程。其它情況下也稱為超雙曲形偏微分方程。不同類型的方程解的形式也不一樣[77]。
用數值方法解偏微分方程時更需要用到矩陣。一個重要的方法是有限元方法,在求解各種物理中遇到的偏微分方程時廣泛使用。有限元方法的基本思想是用一系列「簡單」函數的線性組合來「逼近」偏微分方程的精確解。這些「簡單」函數通常是指將求解區域分割成一定數量的「小塊」後,僅在某一「小塊」上非零的分段線性函數。選定了網格和「簡單」函數後,可以求解關於剛度矩陣的方程得到近似解。有限元理論中證明了在滿足一定的條件下,近似解將隨着網格趨於精細而弱收斂到精確解[78][79]。
概率論中常用到隨機矩陣,即列向量是概率向量(即所有的元素都在0和1之間,並且加起來等於1的向量)的矩陣。隨機矩陣可用來定義有限概率空間中的馬爾可夫鏈。設隨機變量是某個馬爾可夫鏈在時刻的狀態,所有可能的狀態稱為狀態空間,那麼隨機矩陣則記錄了假設已知的可能情況下做各種取值的可能性[80]。的第列第行上的元素表示當的時候,的可能性。的第列記錄了從轉移到各種狀態的可能性。所以叫做時刻的轉移矩陣。如果馬爾可夫鏈的轉移矩陣不隨時刻變化,則稱為齊次馬爾可夫鏈。這時馬爾可夫鏈的吸引態可以通過計算轉移矩陣的特徵向量得到[81]。
統計學中也會用到各種不同的矩陣。描述統計學中常常需要用矩陣的形式來描述數據樣本,顯得更為緊湊。幾個隨機變量的協方差矩陣表示它們之間的協方差關係,在某種程度上表示了它們相互間的關聯程度(但不絕對)[82]。
統計學中用到矩陣的另一個地方是線性回歸中的最小二乘法分析。當觀測到隨機樣本時,線性回歸法的目標是希望找到以下的線性關係:
即將變量表示成的分量的線性組合與一個已知的隨機誤差的和。這個表示可以寫成矩陣的形式,並利用矩陣的奇異值分解來分析[83]。
另一種隨機矩陣(random matrix)是指每個元素都是隨機變量的矩陣,這些隨機變量可以都遵循同一個分布,或各自遵循不同的分布。一個常見的例子是全部元素都是相互獨立的標準正態分布隨機變量的隨機矩陣。這種隨機矩陣在數論和物理中也有應用[84][85]。
線性變換及其所對應的對稱,在現代物理學中有着重要的角色。例如,在量子場論中,基本粒子是由狹義相對論的洛倫茲群所表示,具體來說,即它們在旋量群下的表現。內含泡利矩陣及更通用的狄拉克矩陣的具體表示,在費米子的物理描述中,是一項不可或缺的構成部分,而費米子的表現可以用旋量來表述[86]。描述最輕的三種夸克時,需要用到一種內含特殊酉群SU(3)的群論表示;物理學家在計算時會用一種更簡便的矩陣表示,叫蓋爾曼矩陣,這種矩陣也被用作SU(3)規範群,而強核力的現代描述──量子色動力學的基礎正是SU(3)。還有卡比博-小林-益川矩陣(CKM矩陣):在弱相互作用中重要的基本夸克態,與指定粒子間不同質量的夸克態不一樣,但兩者卻是成線性關係,而CKM矩陣所表達的就是這一點[87]。
1925年海森堡提出第一個量子力學模型時,使用了無限維矩陣來表示理論中作用在量子態上的算子[88]。這種做法在矩陣力學中也能見到。例如密度矩陣就是用來刻畫量子系統中「純」量子態的線性組合表示的「混合」量子態[89]。
另一種矩陣是用來描述構成實驗粒子物理基石的散射實驗的重要工具。當粒子在加速器中發生碰撞,原本沒有相互作用的粒子在高速運動中進入其它粒子的作用區,動量改變,形成一系列新的粒子。這種碰撞可以解釋為結果粒子狀態和入射粒子狀態線性組合的標量積。其中的線性組合可以表達為一個矩陣,稱為S矩陣,其中記錄了所有可能的粒子間相互作用[90]。
矩陣在物理學中的另一類泛應用是描述線性耦合調和系統。這類系統的運動方程可以用矩陣的形式來表示,即用一個質量矩陣乘以一個廣義速度來給出運動項,用力矩陣乘以位移向量來刻畫相互作用。求系統的解的最優方法是將矩陣的特徵向量求出(通過對角化等方式),稱為系統的簡正模式。這種求解方式在研究分子內部動力學模式時十分重要:系統內部由化學鍵結合的原子的振動可以表示成簡正振動模式的疊加[91]。描述力學振動或電路振盪時,也需要使用簡正模式求解[92]。
在幾何光學裏,可以找到很多需要用到矩陣的地方。幾何光學是一種忽略了光波波動性的近似理論,這理論的模型將光線視為幾何射線。採用近軸近似,假若光線與光軸之間的夾角很小,則透鏡或反射元件對於光線的作用,可以表達為2×2矩陣與向量的乘積。這向量的兩個分量是光線的幾何性質(光線的斜率、光線跟光軸之間在主平面的垂直距離)。這矩陣稱為光線傳輸矩陣,內中元素編碼了光學元件的性質。對於折射,這矩陣又細分為兩種:「折射矩陣」與「平移矩陣」。折射矩陣描述光線遇到透鏡的折射行為。平移矩陣描述光線從一個主平面傳播到另一個主平面的平移行為。
由一系列透鏡或反射元件組成的光學系統,可以很簡單地以對應的矩陣組合來描述其光線傳播路徑。[93]
在電子學裏,傳統的網目分析或節點分析會獲得一個線性方程組,這可以以矩陣來表示與計算。
很多種電子元件的電路行為可以用矩陣來描述。設定為輸入向量,其兩個分量為輸入電壓與輸入電流。設定為輸出向量,其兩個分量為輸出電壓與輸出電流。這電子元件的電路行為可以描述為;其中,是2×2矩陣,內有一個阻抗元素、一個導納元素、兩個無量綱元素與。這樣,電路的計算可以約化為矩陣計算。
參見
注釋與參考
外部連結
Wikiwand - on
Seamless Wikipedia browsing. On steroids.