結構相似性
来自维基百科,自由的百科全书
結構相似性指標[1](英語:structural similarity index,SSIM index)是一種用以衡量兩張數碼圖像相似程度的指標。當兩張影像其中一張為無失真影像,另一張為失真後的影像,二者的結構相似性可以看成是失真影像的影像質素衡量指標。相較於傳統所使用的影像質素衡量指標,像是峰值訊噪比(英語:PSNR),結構相似性在影像質素的衡量上更能符合人眼對影像質素的判斷[2][3]。
基本觀念
結構相似性的基本觀念為自然影像是高度結構化的[1],亦即在自然影像中相鄰像素之間有很強的關聯性,而這樣的關聯性承載了場景中物體的結構資訊。人類視覺系統在觀看影像時已經很習慣抽取這樣的結構性資訊。因此,在設計影像質素衡量指標用以衡量影像失真程度時,結構性失真的衡量是很重要的一環。
定義
給定兩個訊號和,兩者的結構相似性定義為:
,
,,。
其中,比較和的亮度,比較和的對比度,比較和的結構(structure),,,,為調整、、相對重要性的參數,及、及分別為和的平均值和標準差,為和的協方差,、、皆為常數,用以維持、、的穩定。
結構相似性指標的值越大,代表兩個訊號的相似性越高。
若使用全等的兩張圖片去做SSIM運算,也就是說且,如此一來
實際使用時,簡化起見,一般會將參數設為及,得到:
。
在計算兩張影像的結構相似性指標時,會開一個局部性的視窗,一般為×的小區塊,計算出視窗內訊號的結構相似性指標,每次以像素為單位移動視窗,直到整張影像每個位置的局部結構相似性指標都計算完畢。將全部的局部結構相似性指標平均起來即為兩張影像的結構相似性指標。
性質
結構相似性指標具有下列性質:
- 結構相似性指標是對稱的,亦即
- 。
- 局限性
- 結構相似性指標的最大值為1,亦即
- 。
- 單一最大值
- 當衡量的兩個訊號完全相同時,若且唯若結構相似性指標的值為1。
- 。
使用
實際使用時,一般會將參數設為及,得到:
。
在計算兩張影像的結構相似性指標時,會開一個局部性的視窗,一般為×的小區塊,計算出視窗內訊號的結構相似性指標,每次以像素為單位移動視窗,直到整張影像每個位置的局部結構相似性指標都計算完畢。將全部的局部結構相似性指標平均起來即為兩張影像的結構相似性指標。
比較
一般認為,結構相似度指標比均方誤差(MS)更適合用來判斷兩張圖像的相似度,原因是結構相似性指標同時考慮圖片亮度、對比與結構資訊,這跟人類的視覺系統的判斷基準較為接近。MSE只考慮兩張圖片的平均亮度誤差而不考慮結構化的資訊,所以當圖片有些微的亮度改變時,MSE改變會很劇烈,但小亮度改變並不會造成人類把兩張圖片判斷成完全不同。
另外一種計算相似度的方法是使用正規化均方根誤差(NRMSE),NRMSE是基於MSE的公式,再除上其中一張圖像的亮度,這樣的處理使得NRMSE對於整體亮度變化比較不敏感,並且讓數值落在比較受限的範圍內以便於比較,然而NRMSE沒有考慮結構化資訊的問題依舊存在。
下面使用了五張相片做實驗,輔助說明:
- Image A:原圖
- Image B:(Image A * 0.5) + 128
- Image C:255 - Image A
- Image D:Image A 疊加影子後的結果
- Image E:對照組

對人類的視覺系統來說,Image A、B、C、D 是一群較為相似的圖片,Image E 則是與其他圖片毫無相關。
這裏是使用Image A跟其他圖片進行相似度的比較,一共使用三種方法:均方誤差(MSE)、正規化方均根差(NRMSE)、結構相似度指標(SSIM)。

然而在上述的比較實驗可以發現,只有結構相似度指標能夠正確反映這個關聯,結構相似性指標在Image B、C、D之中都得到了較高的相似度(其中Image C為負相關),而唯有Image E得到了接近零的相似度。這代表使用結構相似性指標能夠知道Image A、B、C、D之間很相似,而Image E跟其他圖片不相似。反之,MSE與NRMSE皆無法在結果上反映出Image E與其他圖片的差別。
應用
結構相似性指標因其簡單而有效,近年來廣泛被使用在影像與視像處理的相關應用上,例如影像壓縮[4]、影像浮水印[5]、無線視像串流[6]、核磁共振成像[7]等等。
- 相似性評估:
在影像壓縮、影像去噪與影像還原等領域,經常使用結構相似性指標來判斷方法的好壞。以影像壓縮的應用為例,結構相似度用於比較原圖與壓縮後的圖片的差異。原則上希望壓縮後的圖片不會跟原圖差太多,同時壓縮後的圖片又可以節省空間。如果原圖與壓縮後圖片之間的結構相似度是接近1的代表這是個優秀的壓縮方法,反之則是不佳的壓縮方法。 另外,在影像去噪的應用中,有時會採用人工合成的雜訊疊加在圖片上,並且設計去噪演算法去除人工合成上去的雜訊。在這種情況能夠使用結構相似度直接判斷原圖跟去噪後的圖片之間有多接近。結構相似度越接近1,代表去噪演算法能夠成功地把雜訊去除並將原圖還原出來。
- 影像分類:
因為結構相似性設計上盡量貼近人類的視覺系統,所以計算結構相似性的概念還能用於辨識圖片中特定的圖樣。在這類型的應用,通常需要分類演算法對特徵的平移、旋轉與縮放不敏感,所以會使用CW-SSIM取代原本的結構相似度來進行計算。而根據CW-SSIM的作者的描述[8]使用CW-SSIM搭配支持向量機作為分類器,此方法可以在MNIST數據集上達到95%的結果。
限制
結構相似性指標有其限制,對於影像出現位移、縮放、旋轉(皆屬於非結構性的失真)的情況無法有效的運作。為解決此問題,另已發展出在小波域進行運算的結構相似性指標,稱作複小波結構相似性指標[9](complex wavelet SSIM,CW-SSIM)。
當圖片出現平移、旋轉或是縮放時,結構相似度指標會改變得十分劇烈,並很容易將兩張類似的圖片視為不相似的。原因與在計算SSIM時所使用的局部性視窗有關,平移、旋轉或是縮放都會導致視窗內的像素結構完全改變,使SSIM無法正確估計相似度。
下面的實驗是使用Image A 跟Image F、G、H計算相似度
- Image F: Image A 往右平移30像素
- Image G: Image A 逆時針旋轉30度
- Image H: Image A 長寬各縮短6.25%
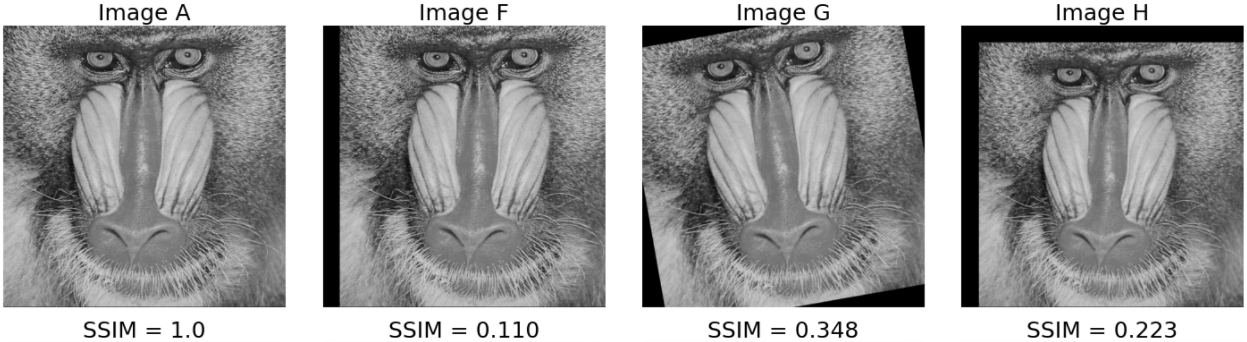
可以看到Image A僅向右移30像素就導致了兩張圖片前後的SSIM為0.11,上述實驗足以說明結構相似性指標的確對這些操作很敏感,要解決這類的問題可以使用複小波結構相似性。
變形
多尺度結構相似性(multi-scale SSIM,MS-SSIM)嘗試去解決結構相似度裏面,依賴特定大小的視窗進行計算的問題,因為如果輸入的圖片的解像度不同,用戶往往需要更改結構相似性的參數,才能得到較合理的結果。多尺度結構相似性,則是把輸入圖片經過若干次的低通濾波器與兩倍下採樣,每次下採樣之後都計算一次結構相似度。最後的結果會同時考量所有不同尺寸的圖片計算出的結構相似性,由此達到指標能自適應不同解像度的圖片的目的。Multi-Scale SSIM Complex Wavelet SSIM 或是 CW-SSIM的作者[10]認為MS-SSIM能得到比SSIM相等或是更好的評量結果。
結構相異性(structural dissimilarity,DSSIM)正好是結構相似性的相反。當兩張圖片相等時,結構相異性為0。反之當兩張圖片很不相似時,結構相異性可以趨近無限大。
另一種結構相異性定義[11][查證請求]為 其中為x與y的結構相似性。當DSSIM越大時,代表兩張圖片越接近,SSIM越接近1,DSSIM的值也越大,當SSIM=1,也就是DSSIM趨近無限時,和原始視訊檔案完全一致。
結構相似性雖然能大致符合人類的視覺系統的感受,但若圖片遇到幾何上的轉換,例如平移、旋轉與縮放時,結構相似性會無法正確描述兩張圖片的相似程度。因此複小波結構相似性(complex wavelet SSIM,CW-SSIM)針對了這個問題進行了改良,CW-SSIM的作者[8]認為與其使用亮度(magnitude)進行比較,不如用相位來進行比較較具有代表性,因此CW-SSIM會在複小波域中計算區域性的相位,並用相位的差異來計算相似性,公式如下:
其中 是複小波轉換後的訊號,而是複小波轉換後的訊號,另外是用於穩定數值的一個常數 如同結構相似性,CW-SSIM的最大值為1,但是不同於結構相似性,CW-SSIM的最小值為0。
參考資料
外部連結
Wikiwand - on
Seamless Wikipedia browsing. On steroids.