Influential observation
Observation that would cause a large change if deleted From Wikipedia, the free encyclopedia
In statistics, an influential observation is an observation for a statistical calculation whose deletion from the dataset would noticeably change the result of the calculation.[1] In particular, in regression analysis an influential observation is one whose deletion has a large effect on the parameter estimates.[2]
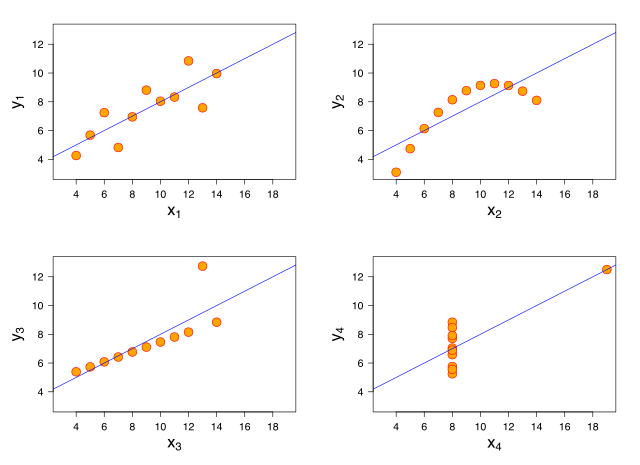
Assessment
Various methods have been proposed for measuring influence.[3][4] Assume an estimated regression , where is an n×1 column vector for the response variable, is the n×k design matrix of explanatory variables (including a constant), is the n×1 residual vector, and is a k×1 vector of estimates of some population parameter . Also define , the projection matrix of . Then we have the following measures of influence:
- , where denotes the coefficients estimated with the i-th row of deleted, denotes the i-th value of matrix's main diagonal. Thus DFBETA measures the difference in each parameter estimate with and without the influential point. There is a DFBETA for each variable and each observation (if there are N observations and k variables there are N·k DFBETAs).[5] Table shows DFBETAs for the third dataset from Anscombe's quartet (bottom left chart in the figure):
x | y | intercept | slope |
10.0 | 7.46 | -0.005 | -0.044 |
8.0 | 6.77 | -0.037 | 0.019 |
13.0 | 12.74 | -357.910 | 525.268 |
9.0 | 7.11 | -0.033 | 0 |
11.0 | 7.81 | 0.049 | -0.117 |
14.0 | 8.84 | 0.490 | -0.667 |
6.0 | 6.08 | 0.027 | -0.021 |
4.0 | 5.39 | 0.241 | -0.209 |
12.0 | 8.15 | 0.137 | -0.231 |
7.0 | 6.42 | -0.020 | 0.013 |
5.0 | 5.73 | 0.105 | -0.087 |
Outliers, leverage and influence
An outlier may be defined as a data point that differs markedly from other observations.[6][7] A high-leverage point are observations made at extreme values of independent variables.[8] Both types of atypical observations will force the regression line to be close to the point.[2] In Anscombe's quartet, the bottom right image has a point with high leverage and the bottom left image has an outlying point.
See also
References
Further reading
Wikiwand - on
Seamless Wikipedia browsing. On steroids.